The Power of AI Labeling Tools in Data Annotation
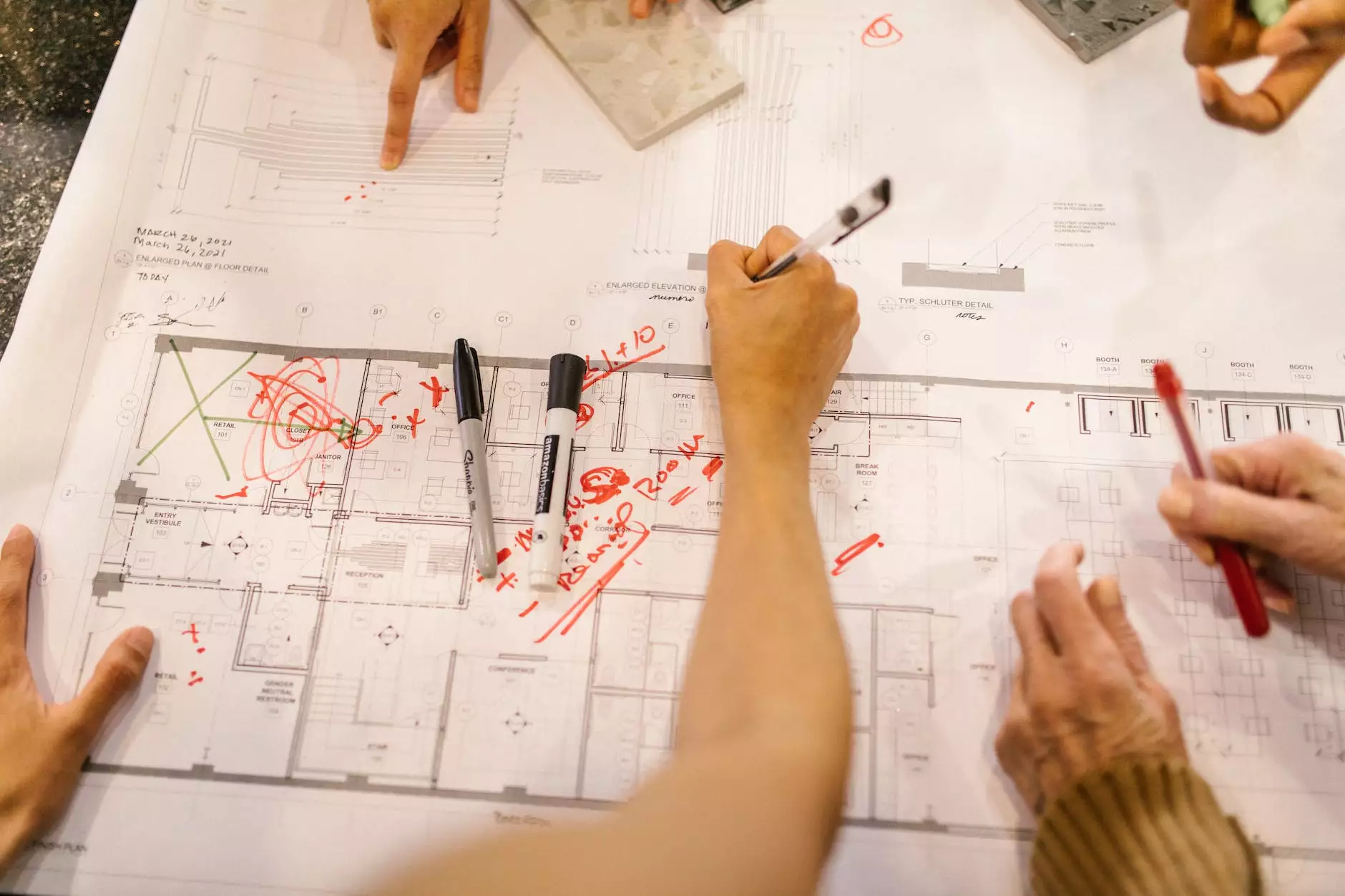
In today's data-driven world, businesses face the enormous challenge of extracting valuable insights from vast volumes of data. One critical component that facilitates this extraction is the use of ai labeling tools. These tools are essential for data annotation, which is the process of labeling data to train machine learning models. In this article, we will explore the significance of AI labeling tools, their applications, and how they can benefit businesses, particularly in the realm of keylabs.ai.
Understanding Data Annotation
Data annotation is the backbone of machine learning and artificial intelligence. It involves the process of annotating data with labels or tags that enable machines to understand the context of the information. This process is crucial for supervised learning, where models learn from labeled datasets, leading to improved accuracy and performance.
The Importance of Quality Data Annotation
Quality data annotation is imperative for several reasons:
- Enhanced Model Accuracy: Properly annotated data ensures that machine learning models are trained on the correct information, leading to better performance.
- Time Efficiency: Efficient data annotation accelerates the training process, enabling faster deployment of models.
- Cost Reduction: High-quality annotations reduce the need for extensive retraining of models, thus cutting down costs.
What Are AI Labeling Tools?
AI labeling tools are software solutions designed to automate the process of data annotation. They leverage artificial intelligence and machine learning algorithms to streamline the labeling process, minimize human error, and improve efficiency. These tools can handle a variety of data types, including images, text, audio, and video, providing versatility for businesses across different sectors.
Key Features of AI Labeling Tools
When selecting an ai labeling tool, it’s essential to understand the key features that can make a difference in your data annotation processes. Here are some critical features to look for:
- Automation: The ability to automate repetitive tasks, significantly reducing the time and effort required for data labeling.
- Collaboration: Tools that allow multiple users to work on the same project simultaneously, enhancing teamwork and productivity.
- Integration Capabilities: Support for integration with existing data pipelines and machine learning frameworks.
- Quality Assurance: Features that help maintain the quality of annotations through validation and review processes.
Benefits of Using AI Labeling Tools for Businesses
Implementing AI labeling tools can bring about significant enhancements for businesses looking to optimize their data management strategies. Some of the key benefits include:
1. Increased Efficiency
AI labeling tools automate routine tasks, enabling teams to focus on more strategic efforts. This efficiency translates into faster turnaround times for projects, allowing businesses to make data-driven decisions more quickly.
2. Cost-Effectiveness
By reducing the time required for data annotation, businesses can see a remarkable drop in operational costs. AI labeling tools can handle large datasets without proportional increases in cost, making them an excellent return on investment.
3. Scalability
As businesses grow, their data requirements change. AI labeling tools offer the scalability necessary to handle increasing volumes of data without compromising on quality or speed. This adaptability is crucial for maintaining momentum in today’s fast-paced business environment.
4. Enhanced Data Quality
With built-in quality assurance processes, AI labeling tools help ensure that the data being annotated meets high standards. This improved data quality leads to more accurate machine learning models and better business insights.
Applications of AI Labeling Tools
The applications of AI labeling tools are vast and span across various industries. Some of the notable areas where these tools make a significant impact include:
1. Healthcare
In the healthcare sector, AI labeling tools assist in annotating medical images for diseases, lab results, and patient histories. This annotated data is vital for developing diagnostic models that can predict health conditions accurately.
2. Automotive
The automotive industry uses AI labeling tools for annotating data from autonomous vehicles. This includes identifying objects, pedestrians, and road signs, crucial for developing safer self-driving cars.
3. E-commerce
In e-commerce, AI labeling tools help categorize products, analyze customer interactions, and optimize recommendations. By properly labeling product images and descriptions, businesses can drive sales and improve customer experience.
4. Finance
In finance, these tools can assist in fraud detection by annotating transaction data and identifying patterns that signify fraudulent activity, thereby protecting businesses and customers alike.
Choosing the Right AI Labeling Tool
With numerous options available, selecting the right ai labeling tool is critical for your business’s success. Here are some considerations to guide your choice:
1. Define Your Needs
Assess the specific requirements of your organization. Determine the types of data you will be labeling, the volume of data, and the desired output. This understanding will help you find a tool that caters to your unique needs.
2. Evaluate Usability
Choose a tool that provides an intuitive user interface, making it easier for your team to navigate and use effectively. A complex tool may lead to longer training times and reduced productivity.
3. Consider Integration
Check if the tool can integrate with your existing systems. Smooth integration allows for seamless data flow and enhances overall efficiency.
4. Look for Support and Community
A robust support system and an active community can be invaluable when you face challenges with your AI labeling tool. Choose a provider that offers comprehensive customer support, documentation, and community forums.
Future of AI Labeling Tools
As the landscape of data continues to evolve, so too will the capabilities of AI labeling tools. Future advancements may include:
- Enhanced AI Algorithms: As AI technology progresses, labeling tools will become even more efficient, with algorithms capable of more accurately annotating complex datasets.
- Greater Customization: Businesses will benefit from tools that offer more customizable solutions tailored to specific industry needs.
- Collaboration Features: Advanced collaboration tools will enable teams across the globe to work together on data annotation projects in real-time.
Conclusion
In conclusion, the role of ai labeling tools in data annotation cannot be overstated. They provide businesses with the power to automate, scale, and enhance the quality of data annotation processes, ultimately leading to better machine learning outcomes and informed decision-making. As industries continue to harness the power of data, the demand for high-quality AI labeling tools is set to grow. Embracing these tools can give businesses a significant competitive edge, allowing them to uncover valuable insights and drive future innovations.